Machine Learning in Health Insurance Verification
Published:
March 29, 2024
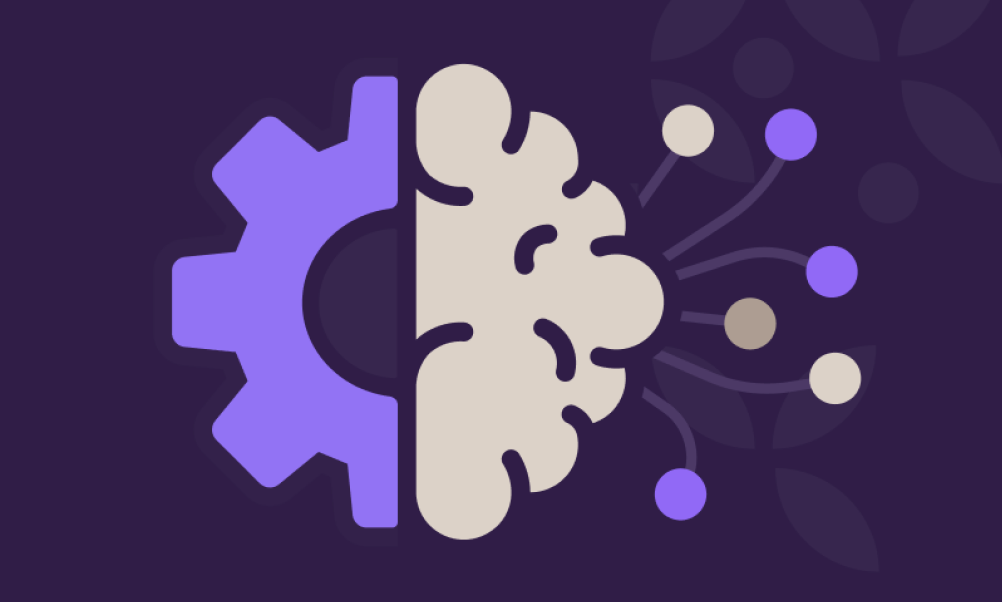
Published:
March 29, 2024
At Nirvana, we believe that machine learning (ML) has a critical role to play in improving healthcare insurance verification and eligibility workflows. Recent developments in Generative AI (Gen AI) and Large Language Models (LLMs) have created a lot of buzz about potential use cases across all industries - however, we believe it’s important to use the right tool for the job, and there remain use cases where a more traditional ML approach is a better fit.
Generative AI is a broader category encompassing various models capable of generating content across different domains like text or images. LLMs are a subset of Generative AI models focused specifically on generating natural language text. An example of an LLM is Open AI’s Chat-GPT.
In short, Gen AI and ML are related concepts, but they refer to different aspects of artificial intelligence.
ML is a concept that encompasses the development of algorithms and models that enable computers to learn from data and improve their performance on a specific task. ML can be categorized into supervised learning, unsupervised learning, and reinforcement learning and it’s a technology that underlies many AI applications.
While LLMs and Gen AI focus on creating specific forms of content (images, text, music, etc.) machine learning focuses on training models to make predictions or decisions based on data.
Now let’s review how these can impact healthcare eligibility and the best use cases.
As it stands today, Generative AI is particularly well suited to three types of tasks: reading, writing, and chatting. The most common traditional machine learning techniques focus on training algorithms to learn from previously labeled data to make a prediction. Here’s a brief overview and the potential healthcare eligibility use cases:
As you can see, when it comes to providing cost estimates for eligibility verification, a traditional ML approach is likely to produce the best outcomes due to its ability to make predictions about patient eligibility.
While LLMs can be a great tool for interacting with patients in a chatbot, reducing the burden on patient support staff, they are not trained to make predictions about a patient’s likely eligibility. And if you’ve spent any time dealing with LLMs, you’ll quickly realize the outputs of an LLM are inconsistent, even when prompting an LLM with the same prompt, the output you receive from the model changes.
We know how important it is for our customers to have reliable cost estimates for each unique insurance plan and that’s why our models and workflows are optimized to produce consistent, accurate cost estimates for our customers. That’s accomplished through training and retaining the ML model we’ve developed.
Regardless of whether you use Generative AI or traditional ML, machine learning cannot guarantee 100% accuracy (what ML practitioners call “non-deterministic”). That’s why at Nirvana, we believe that ML is best used for augmenting billing organizations via AI-assisted workflows as opposed to trying to automate 100% of the eligibility workflow.
Inevitably, one of the major questions we get is, “Will AI and ML replace humans? Will it eliminate jobs?”
The short answer: No.
As any practice or biller will tell you today, much of their time is spent on low-level, repetitive, and time-consuming costs. For organizations, this can lead to cost overruns, the need for additional resources, and a burned-out workforce.
Nirvana can help reduce the workload by automating repetitive tasks which frees billers to focus on more complex tasks that require greater oversight and a deeper understanding of what the patients need. This shifts billers' focus from lower-level activities that a machine would be better suited to, to higher-level activities requiring greater human oversight.
Ultimately, this is all about creating a better system and better outcomes for all involved: billers, the practices, providers, and of course patients. That’s why we built Nirvana. To help practices provide better care by creating a better system through eligibility verification technology.
Nirvana is a healthcare technology company delivering accuracy and reliability in insurance billing through automated patient benefit verification APIs and technology for mental health providers. With Nirvana, healthcare providers receive patient benefit information faster with high accuracy (surpassing human biller accuracy) leading to lower claim denials, less patient churn, and fewer wasted resources. With our unique human-in-the-loop ML process, we’re able to detect sources of denial risks, errors in copayments, coinsurance, and other information that cause downstream surprise bills.
Navigating healthcare coverage and costs doesn't have to feel like wandering in the dark.
We're here to light the way.